Why Arctos Labs Edge Cloud Optimization?
Making distributed clouds more optimal
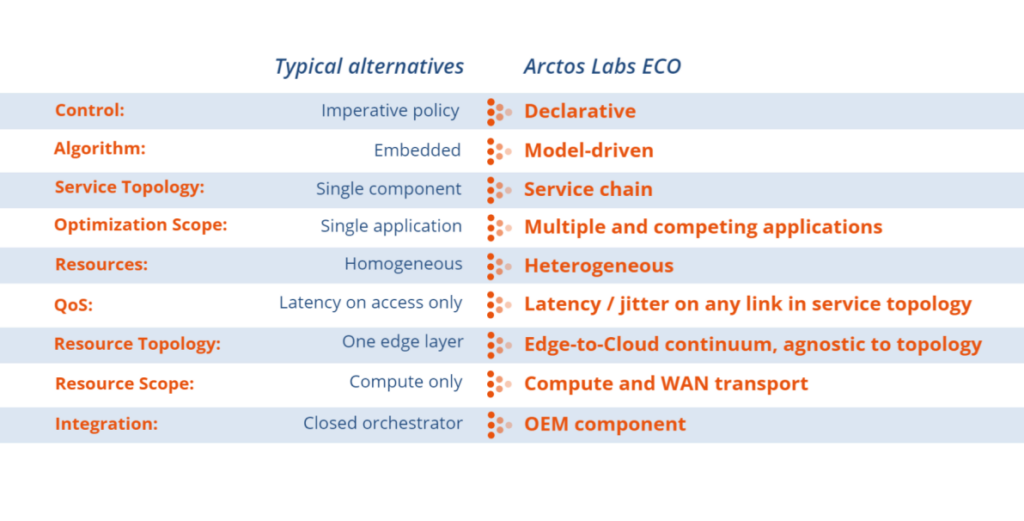
Today’s placement solutions are built from a sequence of filtering. This means that the system removes candidate locations that do not meet specific criteria. This could for example be that locations are not healthy, those that do not have the required capacity, those that do not have the necessary capabilities, or those that are not labeled with required labels.
In many cases the above approach is sufficient, but in other cases, ECO offers a better approach. All of this is under the assumption that a higher degree of automation is needed to combat increasing operational costs.
If anything (or all) of the below fits into your situation, please reach out for a discussion.
Are my application consisting of multiple components with complex interaction?
Traditional placement places each component individually, whilst ECO places what we refer to as a service chain. It means that ECO understands the relationship between the components and that the placement of one constrains the placement of the others. This is especially important when the interaction between the components is subject to high-performance requirements, such as short latency.
If this is the case, then you understand that the individual components cannot be placed randomly and in isolation, they need to be placed as a whole. This is not the same thing as placing all components in one cloud – the amount of data modern applications deal with justifies that some components (but not all) should be deployed at the edge.
Examples: Control-loop systems, Retail
When I have cost vs benefit trade-offs
Many applications are subject to a cost vs benefit trade-off. An optimized placement may impact this trade-off by scaling in/out the number of replicas for certain components and placing them in optimal locations to improve the benefits of the application at the lowest possible cost (i.e. optimize profit)
Such a decision needs a complex mix of metrics that feeds into the optimization algorithm. ECO has an advanced model-driven approach whereby available metrics can easily be combined into a tailor-made algorithm
Examples: SaaS, Application front-ends, Load balancing, Gaming
How can I control how components are distributed? Can’t I use affinity?
Affinity is not a very precise concept, and more importantly, it captures a relation between one component and another. But what if that first component also has a relation to a third component? If both these two relationships are tagged with ”affinity”, the only solution is to place all three components at the same location, which may not be the preferred solution due to other restrictions.
ECO uses a more precise way to capture affinity, by making it possible to describe latency constraints on the links that connect components and map that to the available infrastructure, ECO places components so that the latency constraints are fulfilled.
Examples: Machine control, IIoT
When costs for data transport start to overtake that of computing for my application
Applications are processing increasing amounts of data, and this trend is set to continue. The implication is that our data transport networks risk being overloaded and the costs for such data transport increase for application owners.
Applications that are very data intense need therefore to have some of their components pushed closer to the source of data generation (aka edge). ECO achieves this by cost-optimizing both the compute and data transport parts. This may mean that a more costly compute location may be selected if larger savings can be achieved on the data transport part.
Examples: Video analytics, Artificial Intelligence
When I want to maximize the value of hybrid IT and multi-cloud
Most enterprises are today increasingly interested in Hybrid IT and Multi-Cloud setups. They are complex for two reasons, a) they are heterogeneous by nature, and b) they are distributed. This complexity makes it challenging to automate but also very valuable if you can master the complexity.
ECO supports these scenarios by concluding on the placement of individual application components by using cost models and performance constraints and would therefore provide the best cost vs benefit trade-off and make it easier to utilize new cloud locations when they become available and balance the use of enterprise data centers.
Examples: Cloud bursting, Cloud elasticity
When my application is subject to mobility and fluctuating load
Some applications are accessed from various locations at different points in time. This means that the optimal place for components will be different over time.
ECO supports a dynamic placement process by having the capability to tap into network metrics to understand changes in connectivity and demand density across the network. This capability enables ECO to conclude to move components or scale in/out replicas across the network to achieve needed performance and/or optimize cost.
Examples: Gaming, Logistics, Load balancing
When I have high-availability requirements
When your infrastructure becomes increasingly distributed it imposes a challenge on defining the best remediation to a failure, be it a host, a complete location, or a data transport link failing or predicted to degrade. The number of possible situations just gets out of hand.
This is where ECO can resolve and calculate the best re-shuffle of components to keep as many applications up and running as possible with as good performance as possible.
Examples: SaaS, Application front-ends, Gaming