Arctos Labs Edge Cloud Optimization Solution (ECO)
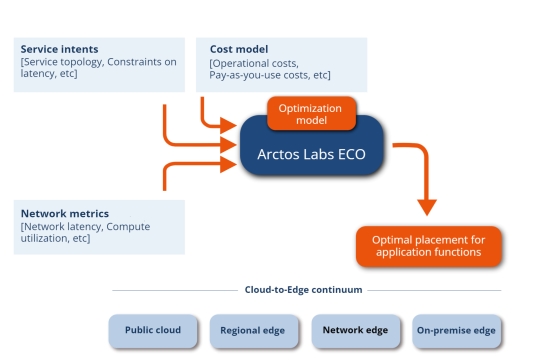
A model-driven optimization engine powered by AI
Optimization is a relative concept! What is optimal in some scenarios may not be optimal in others. This calls for a flexible optimization engine that can be adapted to a range of optimization criteria.
This is why ECO is built on a model-driven approach whereby we can model different optimization scenarios and use code generation and AI technologies to create the executable optimization that runs inside ECO.
It is these advanced technologies that enable ECO to consider multiple services/applications and achieve holistic optimization across Hybrid IT environments and adapt to the ever-changing conditions of a live network.
It further makes it possible to easily change and tailor the optimization formula to consider other input information, to create other optimization output, and most importantly, to change the definition of what is considered optimal
Edge-to-Cloud continuum
One of the key ECO capabilities starts from the fact that most applications are composed of components. These components interact as part of the application as well as with other software entities external to the application. We refer to the structure of the interaction as the service topology.
ECO understands the constraints and requirements of the service topology, referred to as the service intents, and thereby distributes each and every component to match those requirements whilst at the same time applying the optimization criteria to make sure the placement of all components becomes optimal.
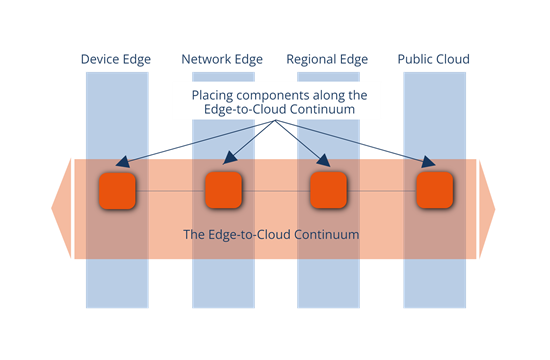
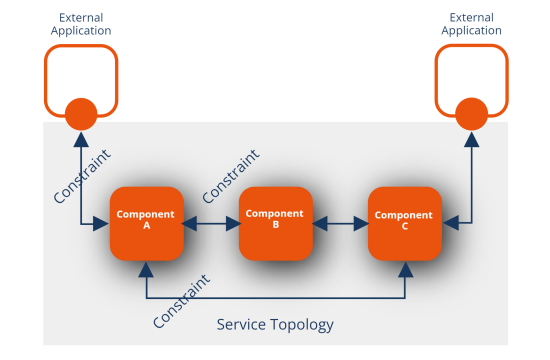
Intent-based placement
Other solutions for placement use various selectors and filters to determine the destination where an application should be placed.
Such expressions rely on that all aspects of placement (now and in the future) are captured by appropriate labels. It is also difficult to take real-time network conditions such as latency into account.
ECO uses an intent-based approach based on expressions of constraints on component interaction, on compute resources, complemented by labels and other properties to determine the best placement.
This approach makes ECO capable to consider real-time metrics as well as dynamically adding compute locations to conduct a holistically optimal placement
Resolve resource competition
Edge computing implies that many compute locations are resource-constrained.
ECO uses service topology constraints to resolve resource competition to move away application components that could be placed elsewhere in case of competition, always applying the optimization criteria.
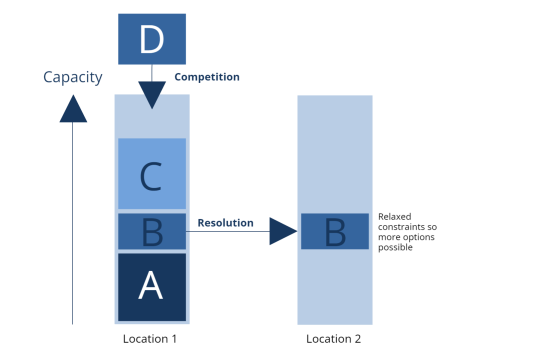
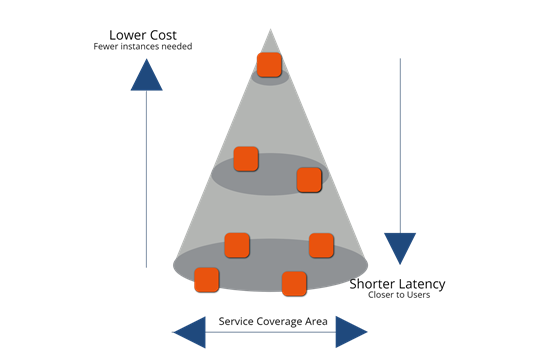
Geo-scaling
An effect of the way ECO models the intents and the service topology is that it enables us to capture intents related to a geographical service area. Combined with intents expressing maximum latency ECO can therefore determine how to optimally scale out an application across the network topology (geography).
It is often more cost-efficient to serve an area from a more central location as that can be done from one component, but it will be at the expense of latency.
In order to cut latency, over the intended service area, components might need to be duplicated at suitable locations yielding a higher cost.
Therefore the cost-performance trade-off is complex and needs to be based on the underlying network metrics to determine how an application needs to be scaled out across the network topology.